02 Jun 2020
Statisticians have developed an online diagnostic system with AI technologies based on COVID-19 chest CT dataset for screening suspected cases
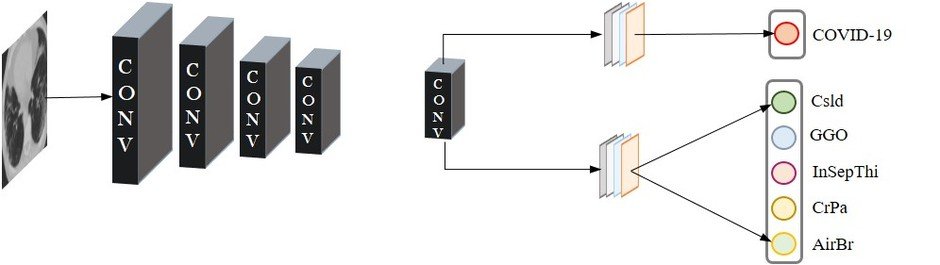
The architecture of the proposed lesion-attention deep neural networks.
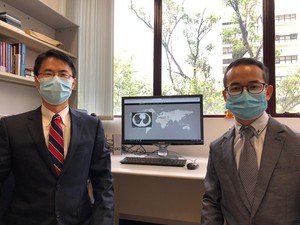
From the left: Professor Guosheng YIN, Head of Department of Statistics and Actuarial Science, The University of Hong Kong, and Dr Bin LIU, Assistant Professor of Centre of Statistical Research, School of Statistics, Southwestern University of Finance and Economics (currently Post-doctoral Fellow at HKU).
A research team led by Professor Guosheng YIN, Head of Department of Statistics and Actuarial Science, The University of Hong Kong, and Dr Bin LIU, Assistant Professor of Centre of Statistical Research, School of Statistics, Southwestern University of Finance and Economics (currently Post-doctoral Fellow at HKU) has been focusing on the related research and study in order to facilitate the testing for COVID-19 based on chest CT scans. A publicly available online COVID-19 diagnostic system has been developed by the team, and this freely accessible diagnostic system would allow individuals to understand the probability of contracting the disease.
The following are the features of the online COVID-19 diagnostic system (https://www.covidct.cn):
- Fast - Require only chest CT image to show the diagnostic result immediately
- Accurate - Accuracy: 88%, AUC (performance measurement for binary classification model): 93%, Sensitivity: 86%, Specificity: 90% (See Note 1)
- Easy to Use - Online web with user-friendly interface
- Open Source - All codes and data are freely available (https://github.com/xiaoxuegao499/LA-DNN-for-COVID-19-diagnosis)
With years of research experience in Biostatistics and Clinical Trials, Professor Guosheng Yin and his team have been actively extending the applications of AI technologies to medical fields in recent years. Meanwhile, the use of chest CT scans for screening suspected cases has been common in the research study of different diseases.
“The main reason that we decided to perform the diagnosis based on chest CT scans is because we have accumulated research experiences in the field of Computer Vision. Moreover, there are still many issues with the RT-PCR testing for COVID-19 in terms of false negatives and time lag for diagnosis. The RT-PCR testing usually takes a swab from nose or throat where the coronavirus may gather, and sometimes it requires several tests to make a final confirmation. This would put patients at a great disadvantage, as they cannot be diagnosed in a fast way and be provided with the necessary quarantine and treatment at an early stage.” said Professor Yin.
As discovered in radiological research, CT scanning may serve effectively in testing for COVID-19, particularly amongst those with no symptom or minimal symptoms. “This is because the coronavirus will typically first attack the lungs and cause lesions after it enters the body. Therefore, by integrating AI technologies, we can make use of the patients’ chest CT images for early diagnosis. However, since most of the chest CT datasets of COVID-19 patients are not publicly shared, we need to spend much time to search for publicly available samples and tag them,” added Professor Yin.
Building this digital platform substantiates again that Radiography and Computer Vision can be perfectly integrated, actualizing the practical use of AI technologies in medical fields. In the early study of CT scanning for COVID-19 diagnosis, the prediction as published in some of the peer researchers’ papers could not achieve the clinical standards. It is believed that, besides a small sample size, it is mainly because the rich annotations associated with the CT images have not been fully utilized. The major difference between this batch of CT images and the traditional medical imaging dataset is that each of the CT samples is collected from a research preprint. In these papers, clinical experts have comprehensively annotated the chest CT images of the COVID-19 patients with detailed lesion descriptions. By leveraging on these text reports from 760 research papers, the research team at HKU has further analyzed and pinpointed five different lesions in association with COVID-19 and each of the confirmed patients is identified with at least one of the five lesions or more. These five lesions are the distinctive features that differentiate COVID-19 from the general pneumonia or other lung diseases. In this regard, the research team at HKU has designed a lesion-attention deep neural network (LA-DNN) model based on the CT images. Whilst the proposed data-driven LA-DNN model focuses on the primary task of binary classification for COVID-19 diagnosis, an auxiliary multi-label learning task is implemented simultaneously to draw the model’s attention to the five lesions of COVID-19. As both tasks are trained synchronously while it shows that the auxiliary task promotes the primary task to focus its attention on the lesion areas and, as a result, the diagnostic accuracy of COVID-19 can be improved drastically.
After launching the online COVID-19 diagnostic system, the research team will continue to collect new samples and improve the training model periodically. With regard to the future direction of the applied work, Professor Yin and Dr Liu said, “We hope that medical staff battling with the disease can make use of the system and share their local patients’ image data, in order to initiate collaborative research and accommodate the urgent demands for COVID-19 testing. At the moment, most of the research papers do not share the data and the computer codes, and this does not facilitate knowledge exchange and disease prevention around the globe, yet our online system, data and computer codes are all publicly and freely available for everyone in the world.”
Note 1:
Sensitivity and specificity are terminologies used in medical diagnosis. Sensitivity, the so-called true-positive rate, measures the percentage of actual positives which are correctly identified. The larger the sensitivity, the better the diagnostic testing for identifying patients. Specificity, the so-called true-false rate, measures the percentage of actual negatives which are correctly identified. Likewise, the larger the specificity, the better the diagnostic testing for confirming negative cases. With regard to COVID-19, we emphasized sensitivity more in order not to misidentify any real COVID-19 patients.