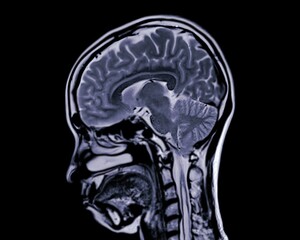
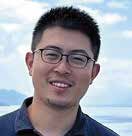
Collaborator // City University of Hong Kong
Medical imaging analysis plays a central role in modern medicine. With the progression of imaging technologies, such as CT, MRI, fMRI, the diagnosis and treatment of diseases have experienced significant improvements. Although image analysis has been intensively studied over the past years, medical image data differ from general images in two main aspects. First, medical imaging typically has a much smaller sample size, but with higher order and dimension. Second, while predicting the outcome is a top priority in many image recognition problems, medical imaging analysis places greater importance on interpreting the model. As a result, popular image analysis methods such as convolutional neural networks can be hardly applied to medical imaging data.
Our researcher introduces an innovative statistical learning method for medical imaging analysis that can adapt to low sample size limitation, enjoy good interpretability and achieve desired prediction power. This approach not only offers a compelling statistical perspective on medical imaging analysis but also highlights the synergy between classic statistical methods and contemporary AI challenges.
醫學影像分析在現代醫學中起扮演着關鍵角色,尤其在CT、MRI、fMRI 等影像的快速發展下技術的進步,疾病的診斷和治療效率得到了顯著提升。我們的研究人員提出了一種創新的統計學習方法用於醫學影像分析。該方法不但能適應醫學數據中小樣本量的限制,且具有良好的解釋性和理想的預測效果。這除了為醫學影像分析提供了獨特的統計視角,亦展示了經典統計方法與現代人工智能共同發展的無限可能性。
Journal paper: Deep Kronecker Network (published in Biometrika, 2024)
< Previous | Next > |